Sensors and AI for factory automation
By Dr. Ola Friman Head of Research & Development Machine Vision, Leader I4.0 Deep Learning Initiative, SICK AG
Saturday, 01 July, 2023
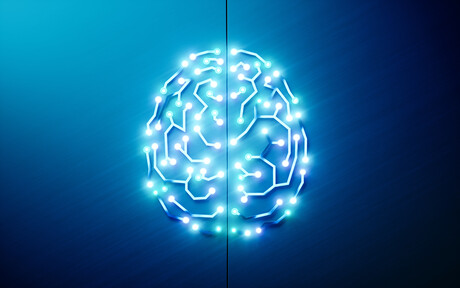
Machine Learning methods based on Deep Neural Network models have in recent years made breakthrough strides in automated perception of data, e.g., for interpretation of images, speech and text. Whereas research results hold a promise of the next leap in automation levels there are also challenges concerning data, engineering, processes and communication that are important for successful adoption. Addressing these challenges will be key to get past technology pilot phases into operational deployments.
Industrial factory automation relies on sensors such as cameras, LiDAR, light grids, RFID and encoders to provide the perception capabilities necessary for decision-making and control, e.g., for sorting, robot picking and quality inspection. Computational algorithms broadly referred to as Artificial Intelligence (AI), process the raw sensor data to extract relevant information and to form decisions. Traditional algorithms consist of rules and mathematical operations designed and parametrized by human experts. A simple example application is to discard a produced item if a hole dimension is not within a given tolerance threshold, where both the mathematical operations to extract the hole dimension from an image and to set the tolerance threshold value are design questions for human domain experts.
Machine Learning offers a different algorithmic approach to the above inspection problem in which the human handcrafting is replaced by an optimization of the parameters in a Machine Learning model that maps the raw sensor data as input to the desired output decision to reject the item or not. What is ultimately left for the human is to give examples of correct mappings, i.e., to supply training images of holes with the right and wrong dimensions respectively. One advantage of the Machine Learning approach is that the underlying mathematical optimization procedures can handle millions of model parameters, which is impossible to handle for a human. It can thereby also find solutions that are not obvious to a human. A consequence of this advantage is however that the Machine Learning solution often becomes a black box where the inside decision mechanisms cannot be understood, with consequences for life cycle management and general trust in the system.
In recent years, the use of so-called Deep Neural Network models have been shown to outperform human handcrafted algorithms within the machine vision and speech understanding domains. For factory automation, one application of Deep Neural Networks is to mimic the outstanding human visual perception.
This is achieved by optimising the neural network to reproduce human responses to visual data for tasks such as visual defect inspection, localizing objects in the camera field-of-view, sorting based on visual appearance or spotting foreign items in food production. In parallel and in conjunction with the advancement in AI technology, related disciplines also experience strong development, including robotics, data connectivity, Internet of Things, miniaturization of computing power and cloud technology. This paves the way for the next generation digital transformation manufacturing systems with a high degree of automated and optimized decision-making, leading to improved production flexibility, resource utilization, waste minimization and product quality. This paper discusses opportunities and challenges for the next decade 2020–2030 related to sensor development and AI in form of Deep Neural Networks towards digital transformation production systems. The following sections highlight opportunities and challenges in adopting the Deep Neural Network technology for factory automation.
Opportunity 1: Sensor perception
The most obvious way Deep Neural Networks may contribute to more efficient production processes is by automating tasks that have not been tractable by means of conventional algorithms. Until now, such tasks either have required the interpretation skills of a human or were simply not possible at all.
Opportunity 2: Measurement utilisation
While predictions around AI typically often revolve around solving new automation applications, an overlooked aspect is to utilize improved perception skills to simplify existing applications by a more efficient utilization of the measurement data. A straightforward example would be to replace high-resolution 2D cameras with lower resolution ones, but one can also foresee examples where a 2D camera plus improved AI can accomplish the same task as a larger 3D camera. Trends in this direction can be seen within the robotics domain where Deep Neural Networks trained on CAD models can estimate the six-dimensional pose, 3D location and 3D orientation, of an object from a 2D image of it. A practical consequence may be that one can make lighter and small-sized sensor solutions fitting more narrow spaces.
Opportunity 3: A new configuration paradigm A key property of Machine Learning and Deep Neural Network approaches is that they are configured in a fundamentally different way compared to a traditional algorithmic approach, i.e., through a well-defined procedure from collecting the raw data, annotate the raw data, train and finally deploy a neural network.
For more information on Deep Learning capabilities in food manufacturing and distribution applications please contact SICK Australia and New Zealand.
Driving the Food Transformation Together with Grundfos
The food and beverage industry is in the middle of a transformation driven by the need for more...
Enhancing Food Safety: Digestion of Seafood Samples with Multiwave 7000 with PDC (Pressurised Digestion Cavity) for Optimising Mercury Analysis in Seafood Samples
In the realm of food safety, the issue of mercury concentration in seafood looms large, demanding...
Automating Original Gravity measurement in beer making
FLOWave — an acoustic multiparameter flowmeter from Bürkert — allows brewers to...